Since the term was coined, DataOps has expanded the way that people think about data analytics teams and their potential. 2020 was a huge year in DataOps industry acceptance. Media mentions of DataOps are on track to increase 52% over the prior year. To date in 2020, DataKitchen has seen an additional 5K downloads of the DataOps Cookbook. Industrywide, searches on DataOps (and derivatives) are up 500% over the past three years. Gartner analyst inquiries related to DataOps are up over 1000%.
Looking ahead to 2021, we see signs of DataOps adoption in large enterprises, expansion of DataOps into new domains, new players, and a radical rethinking of how work gets done in data organizations. Here then are our top six DataOps trends for the year 2021.ย
Enterprise DataOps Transformation
We started tracking the DataOps enterprise software ecosystem back in 2017. Today there are over 75 vendors of enterprise software that market a tool addressing some aspect of DataOps. As the maturity of the ecosystem grows, enterprises have begun to struggle with implementation, asking questions such as how to choose a first DataOps project, how to formulate a long-term DataOps strategy, how to assess DataOps organizational readiness, and how to structure a DataOps team and organization. These challenges are a natural part of any transformative methodology that demands a complete rethinking and replanning of workflows. In 2021, we will see a rise in services that address these concerns. The early adopters have crossed the DataOps chasm and can offer guidance to the early majority. DataOps experts will offer services that enable and support Enterprise DataOps Transformation that automates workflows and relieves bottlenecks associated with analytics development and operations.
Data Observability
Complex data operations pipelines integrate data from dozens or hundreds of disparate sources. Data then traverses data centers, toolchains, and distributed teams on its way to producing value for the enterprise. The advent of self-service analytics tools adds another dimension to analytics workflows with ad hoc data pipelines instantiated on-demand. The evolving topologies that comprise data operations pipelines present daunting challenges. How can the data team prevent non-conforming data or other errors from corrupting the analytics? Enterprise-critical analytics serve as the backbone of operations. In 2021, we anticipate broad investment in data observability as companies leverage automation to address the mammoth challenge of determining the health of centralized and decentralized data pipelines. Data observability integrates testing, monitoring, and real-time alerts into data pipeline orchestration so that data organizations have an ability to view data operations at-a-glance and optionally drill down into the details.
DataGovOps
Busy data scientists sometimes treat governance as an afterthought. With the pressure to cope with an endless stream of analytics updates and the exponential growth of data, there isnโt always time to update and maintain the business glossary, data catalog, and data lineage. Data quality assessments are important, but are incredibly time-consuming and resource-intensive and when complete, reflect only an outdated snapshot in time. Governance is the next major domain ripe for DataOps automation. In 2021, companies will begin embracing DataGovOps (governance-as-code), the idea that governance can be implemented as a set of automated workflows that execute as part of analytics continuous deployment.
A prime example of DataGovOps is DataOps โKitchensโ โ self-service analytics development environments created on-demand with built-in background processes that monitor governance. If a user violates policies by adding a table to a database or exporting sensitive data from the sandbox environment, an automated alert can be forwarded to the appropriate data governance team member. The code and logs associated with development are stored in source control, providing a thorough audit trail. DataGovOps actively promotes the safe use of data with automation that improves governance while freeing data analysts and scientists from onerous manual tasks.
Rise of the DataOps Engineer
As 2020 stretches into 2021, expect to see increased attention on the DataOps engineer, a relatively new role in many data organizations. Following in the footsteps of DevOps Engineers in the software industry, we predict that the DataOps Engineer will become one of the most sought-after and highly compensated members of the data analytics team.
DataOps Engineers implement the continuous deployment of data analytics. They give data scientists tools to instantiate development sandboxes on demand. They automate the data operations pipeline and create platforms used to test and monitor data from ingestion to published charts and graphs, promoting observability. Through tools automation, the DataOps Engineer eliminates data lifecycle bottlenecks, which sap data team productivity. A DataOps Engineer who understands how to automate and streamline data workflows can increase the productivity of a data team by orders of magnitude.ย
Self-Service Sandboxes
The key to maximizing the productivity of data scientists is to alleviate the bottlenecks in their development workflows. For example, it may take 10-20 weeks for a data scientist to create a development environment when a project begins. We envision a trend in 2021 toward automation of self-service sandboxes that reduce the time frame for environment creation from months to days/hours. We call these on-demand environments โKitchensโ and they include everything a data analyst or data scientist needs in order to create analytics: complete toolchain, reusable microservices, security, prepackaged data sets, integration with team workflows, continuous deployment automation, observability, and governance. The environment is โself-serviceโ because it can be created on demand by any authenticated user. Looking forward, enterprises that stubbornly insist on creating environments manually, will find themselves unable to match the agility of competitors.
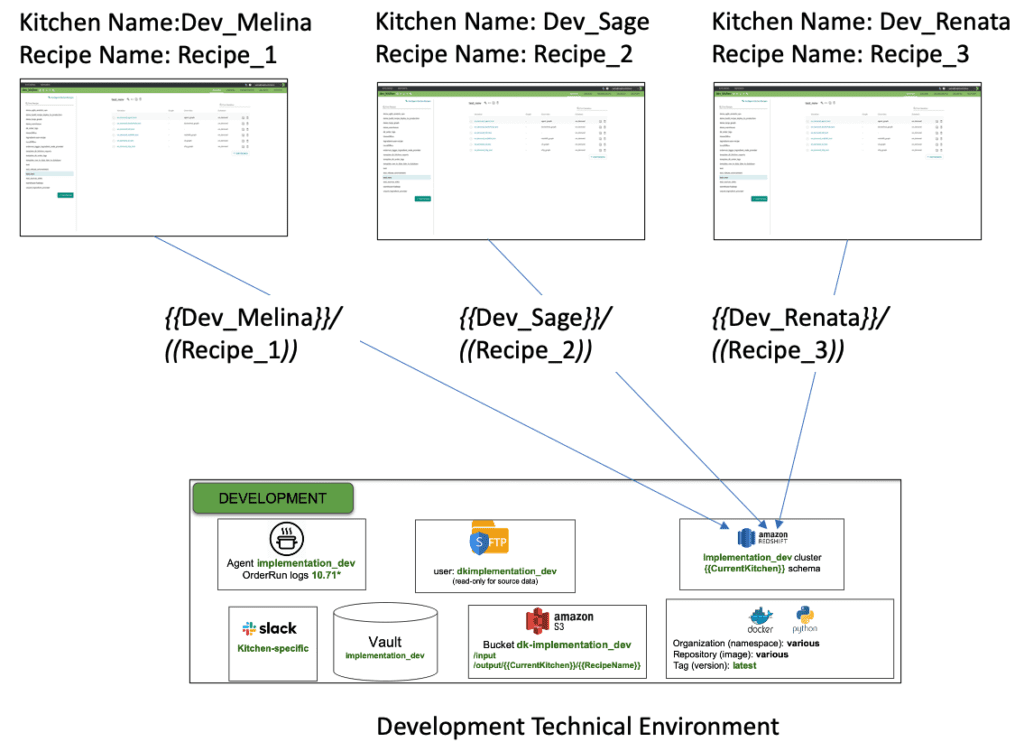
Kitchens include everything a data analyst or data scientist needs in order to create analytics: complete toolchain, reusable microservices, security, prepackaged data sets, integration with team workflows, continuous deployment automation, observability, and governance.
ย
Expansion of the DataOps Tools Landscape
Some organizations try to implement DataOps from scratch using DevOps and workflow tools, however, this approach overlooks the unique requirements of data analytics automation: management of the data factory, creative managed-chaos of self-service analytics, wide divergence in toolchains, meta-orchestrations spanning toolchains, complexity in dev environments, data observability, and the predisposition against software complexity among data professionals. Expect 2021 to bring new DataOps tools and solutions to the market that address the unique needs of data scientists and analytics creation. We expect to see DataOps solutions attracting unprecedented venture investment and existing players adding features that tailor their offering to the data analytics market. Should be fun to watch.
2020 was a tumultuous year. Strategic plans that were approved in late 2019 had to be scrapped amidst tremendous economic uncertainty. As data professionals, we know that data-driven decision making is now more critical than ever. Data analytics can serve as a companyโs most valuable tool when navigating a rapidly evolving environment. Data-analytics teams are one of a companyโs most valuable assets. DataOps helps data organizations increase productivity, improve responsiveness, and deliver insights that shed light on the challenges ahead.