Industry analysts who follow the data and analytics industry tell DataKitchen that they are receiving inquiries about โdata fabricsโ from enterprise clients on a near-daily basis. Forrester relates that out of 25,000 reports published by the firm last year, the report on data fabrics and DataOps ranked in the top ten for downloads in 2020. Gartner included data fabrics in their top ten trends for data and analytics in 2019. From an industry perspective, the topic of data fabrics is on fire.
What is a Data Fabric?
Whenever a new technology or architecture gains momentum, vendors hijack it for their own marketing purposes. This is happening to the term โdata fabric.โ Tools vendors are creating their own definitions of โdata fabricโ to promote their own product and solution offerings. If you search the Internet for a definition of data fabrics you can see discussions of storage, AI augmentation, and other tools. It is also difficult to understand a new technology when people discuss it in terms of its benefits. For example, one could say that data fabrics address complexity, eliminate silos, unify toolchains and help organizations derive insights. Thatโs all justifiable, but the same things could be said of other technologies, so it doesnโt actually explain what data fabrics are and what they do.ย
Data organizations are buckling under the strain of numerous data pipelines acting on large, complex, and distributed data sets. Data fabrics purport to offer a unified approach to manage the cacophony of heterogeneous toolchains being thrown at data problems. Data fabrics are best regarded as an architecture or design concept, not a specific set of tools.
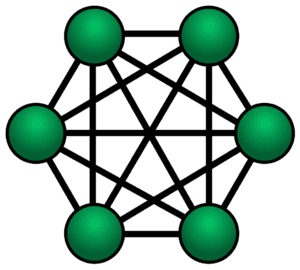
Figure 1: A โfabricโ denotes point-to-point connectivity between all nodes
The term fabric gets its name from an architectural approach that provides full point-to-point connectivity between nodes. In Figure 1, the nodes could be sources of data, storage, internal/external applications, users โ anything that accesses or relates to data. Data fabrics provide reusable services that span data integration, access, transformation, modeling, visualization, governance, and delivery.ย In order to deliver connectivity between all these different services, data fabrics must include connectors to data ecosystem tools.
A cynic could argue that data fabrics retrace many points from the discussion on data virtualization that began a decade ago. Data fabric enthusiasts assert that the design pattern is much more than that and reference one or more emerging data analytics tools: AI augmentation, automation, orchestration, semantic knowledge graphs, self-service, streaming data, composable data analytics, dynamic discovery, observability, persistence layer, caching and more. Data fabrics seek to harmonize all of these diverse technologies and tools โ which ones depend on who is doing the talking. Some thought leaders are saying that enterprises need to implement data fabrics in order to work in an agile, customer-focused way. Data fabrics can add value, but they are not a panacea. Itโs important to understand that migrating to a data fabric is not guaranteed to improve business agility.
Process Constraints
Letโs use an analogy. When you drive down Massachusetts Avenue in Cambridge, Massachusetts you hit a stoplight every few tenths of a mile. This goes on for miles. If a Ferrari Enzo and a Honda Civic raced from Cambridge to Arlington during rush hour, stopping at stoplights and observing traffic laws, itโs a fair bet that they would arrive at around the same time. Maybe the Ferrari would zip ahead at times, but between stoplights and long lines of traffic, the inherent advantages in the Ferrari design would be handicapped or perhaps even totally neutralized.ย
The point is that analytics agility is more about removing obstacles and process bottlenecks than optimized tool performance. The people that data organizations hire and the tools that they buy are Ferraris. Then they place them on busy boulevards wading through long lines of traffic, waiting at stoplights, and force them to observe speed limits. Data fabrics are the latest example of putting a Ferrari on Massachusetts Ave.
The stoplights in data analytics are all of the obstacles that degrade productivity and agility: data errors, manual processes, impact review, lack of coordination, and general bureaucracy. An organization that takes seven weeks to create an analytics development environment will still take seven weeks, even after they spend millions of dollars implementing a data fabric. Tools are sexy, but you know what is really sexy? Turning data analytics into a sustainable competitive advantage because your data team can go really fast while incurring virtually zero errors. How do you create the most agile data analytics team in your industry? You start by looking at your processes and workflows.
Start with a DataOps Process Fabric
Data fabrics can be helpful, but we think it is much more effective to start with a โprocess fabric.โ A process fabric begins with a DataOps superstructure that connects with all your existing tools and orchestrates your data pipelines and workflows (Figure 2). A DataOps process fabric connects your people, processes, and tools together in a giant web of interconnectedness.ย Once you have that in place, you can start alleviating bottlenecks. If you have data errors that drive unplanned work, then orchestrate a battery of statistical and process controls that qualify data sources and data processing. If it takes weeks to create development environments, then use automation and orchestrate the creation of environments on-demand. Take control of your data factory with process orchestration. With fewer traffic lights, your Ferrari tools and people will accelerate into an open road.
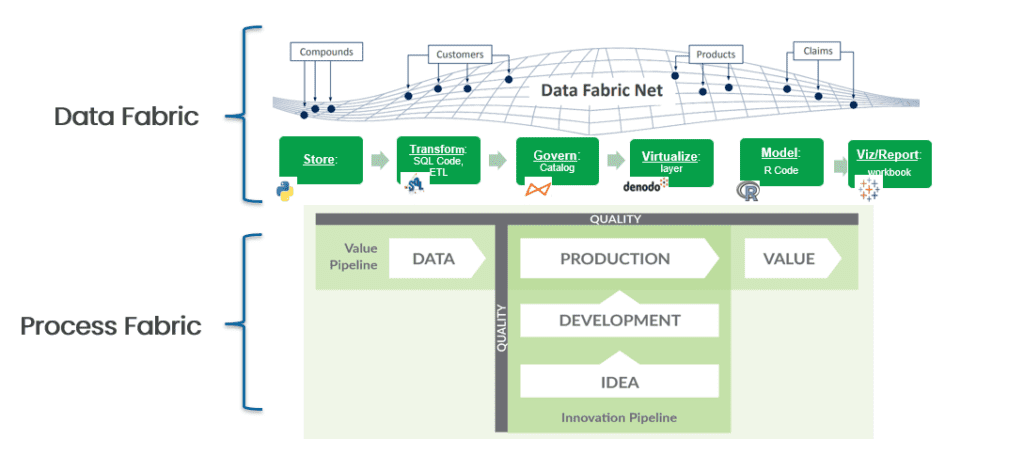
Figure 2: A process fabric begins with a DataOps superstructure that connects your existing tools and orchestrates your data pipelines and workflows.
A DataOps process fabric unifies your various toolchains into a hierarchy of observable orchestrations that we call meta-orchestration. Once that is done, your teams can focus on their specific local orchestrations without struggling with all of the task coordination that makes inter-team collaboration challenging. A process fabric unifies your existing tools and provides the platform on which you can iterate toward alleviating bottlenecks. You may find that technologies like data virtualization, augmented data catalogs, or some other components associated with data fabrics are helpful. The DataOps process fabric gives you control over your technology roadmap so you can evolve incrementally in ways that make sense given your goals and constraints. While some people see data fabrics as an ideal path to DataOps, we think the opposite is more compelling. A DataOps process fabric is a great way to begin a smooth evolution towards a data fabric.
The DataKitchen DataOps Platform provides the toolchain superstructure, meta-orchestration, observability, and environment automation that can help you identify and address process and workflow bottlenecks. If analytics agility is the goal, then process-focused optimization should drive tools decisions and a DataOps process fabric should drive evolution to data fabrics or other technologies that put your Ferrari data team on the fast track.