The Challenge
A large pharmaceutical Business Analytics (BA) team struggled to provide timely analytical insight to its business customers. The company invested significant effort into managing lists of potential prescribers for certain drugs and treatments. However, the BA team spent most of its time overcoming error-prone data and managing fragile and unreliable analytics pipelines.ย
Customers and market forces drive deadlines and timeframes for analytics deliverables regardless of the level of effort required. It may take six weeks to add a new schema, but the VP may say she needs it for this Fridayโs strategy summit. The BA team was under constant pressure to deliver analytics on demand.
The BA team often did not receive a data platform tailored to their needs. IT gave the business analysts a bunch of raw tables, flat files and other data. What they received from IT and other data sources were, in reality, poor-quality data in a format that required manual customization. Out of hundreds of tables, business analysts had to pull together the ten tables that mattered to a particular question. Tools like Alteryx helped a little, but business analysts still performed an enormous amount of manual work. When the project scope and schedule were fixed, managers had to add more staff to keep up with the workload.ย
If the IT or data engineering team did not respond with an enabling data platform in the required time frame, the business analyst performed the necessary data work themselves. This ad hoc data engineering work often meant coping with numerous data tables and diverse data sets using Alteryx, SQL, Excel or similar tools. Business analysts were living under constant pressure to deliver. They spent 80% of their time performing tasks that were necessary but far removed from the insight generation and innovation that their business customers needed.
The dynamic nature of data leads to complexity, including significant quality control (QC) challenges. Testing and validating analytics took as long or longer than creating the analytics. QC is extraordinarily time-consuming unless it is automated. Business analysts needed the flexibility that comes with control of data and orchestrations. Management of the process can’t be performed โoff the gridโ without control, enforcement and consistency.
The Solution
To meet the demand for continuous, high-quality insight, the BA team implemented a DataOps โprocess hub.โย This allows the Data Analysts to take control of the workflows that operate on and around data.ย A process hub ensures that the processes and workflows that create the enterprise data platform are just as important as the data itself. Some data analysts use long checklists to execute steps that integrate, clean and transform data. A process hub automates, synthesizes and synchronizes these steps, so they do not have to be executed and managed manually.
Workflows that add value to data are critical elements of intellectual property that must be retained and controlled. A DataOps process hub instantiates all processes and workflows (i.e., tribal knowledge) related to data and analytics into code. With a process hub, workflows are curated, managed and continuously improved. A robust and vibrant process hub is a clear sign of data organizational maturity.ย
The process hub manages significant complexity because data flows in many directions between platforms, tools, people and functions. As data keeps changing, the process hub enforces quality and the functional order of operations. Valid data depends on a robust, repeatable process. The process hub catches and manages data and analytics errors. Without the process hub, errors cause outages. There is no โsingle version of the truthโ without a โsingle version of the process.โย
This large enterprise has many products and brands with overlapping marketing campaigns. The process hub leverages the work product of several operational systems depicted on the right side of figure 1: a master data management (MDM) system, opt-out management from active marketing campaigns, a CRM and a marketing campaign management platform. These systems interface to the process hub through automated orchestrations. If data is sequestered in access-controlled data islands, the process hub can enable access. Operational systems may be configured with live orchestrated feeds flowing into a data lake under the control of business analysts and other self-service users.
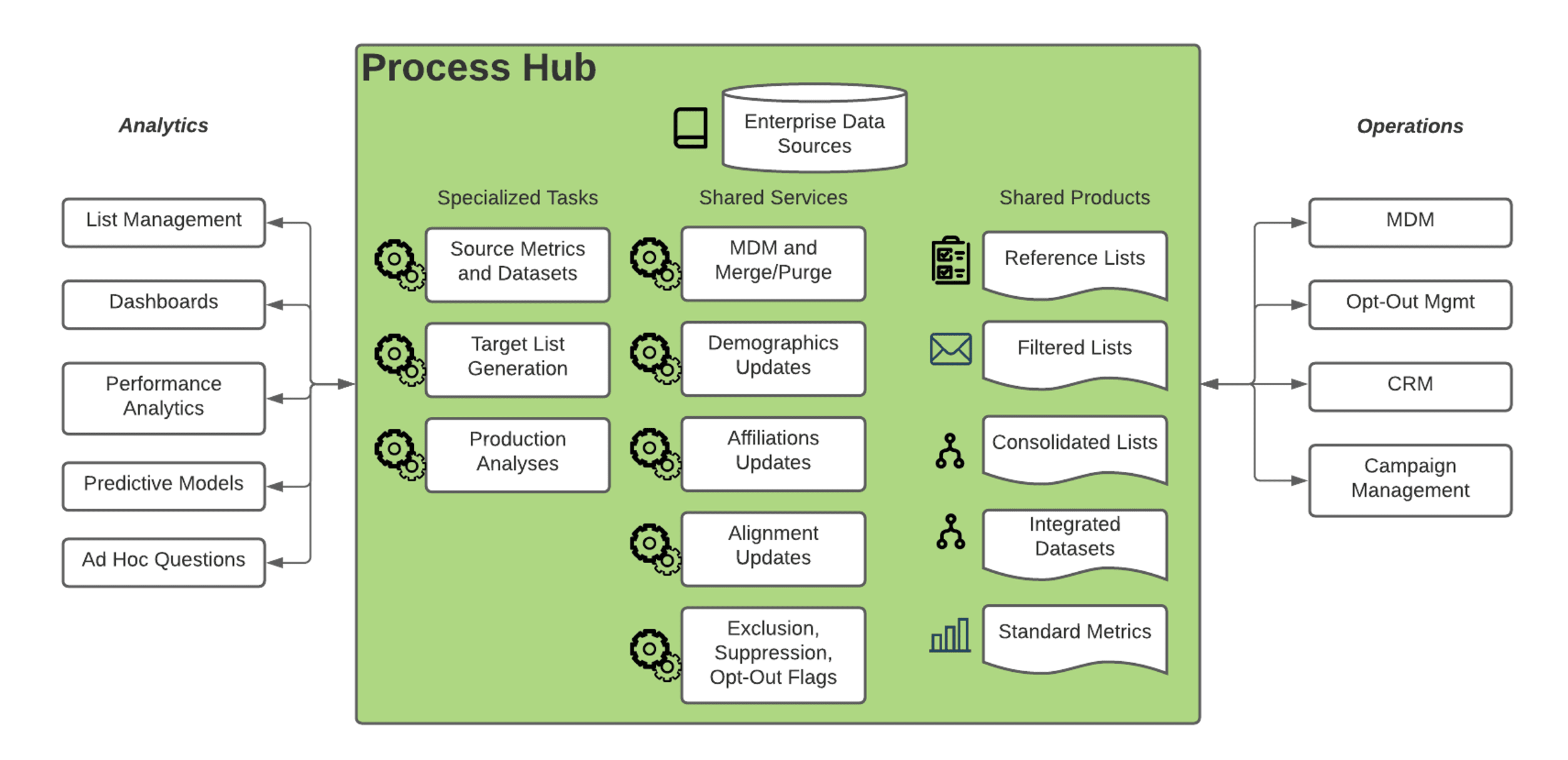
Figure 1: A DataOps Process Hub
The process hub provides shared services and resources that feed the needs of the enterprise, divisions, product teams and specific projects while also providing feedback to operational systems. On the left side of figure 1, we see analytics produced for the various business development stakeholders. Despite the complexity, mission-critical analytics must be delivered error-free under intense deadline pressure.
The business analysts creating analytics use the process hub to calculate metrics, segment/filter lists, perform predictive modeling, โwhat ifโ analysis and other experimentation. Requirements continually change. Data is not static. Data workflows are not like IT infrastructure that is set up and then simply maintained. New analytics are added, and existing analytics are refined. The process hub resynchronizes master data, updates demographic information and reapplies suppressions, opt-outs and other attributes.
The Results
With the DataOps process hub in place, the BA team is significantly more productive.ย The process hub lowers the cost per additional data and analytics question. It centralizes the process so that it can be generalized and then applied to specific analytics questions in a fraction of the time it would otherwise require. An automated, governed, and reusable process decreases unplanned work and reduces the cycle time of change. It also minimizes the need for outside consultants who tend to rely upon heroism and tribal knowledge. A process hub is the key to creating an open, secure platform that enables those with access to efficiently update and skillfully manage the processes used to create data and analytics.ย
Bottom line, the process hub enabled the company to deliver higher quality analytics at a faster rate with fewer business analysts.ย They experienced:
- Delivery of new analytics in one-third the time;
- Data errors reduced by 80%;
- 5X increase in the number of sales and marketing users supported per data analyst; and
- Visualizations updated per week increased from 50 to 1500
The process hub helped this pharmaceutical company achieve its strategic goals by improving analytics quality, responsiveness, and efficiency. The process hub supports improved processes, automation of tools, and agile development of new analytics. With a process hub, the analytics team delivered value to users in record time, accelerating and magnifying their impact on top-line growth.
For more on process hubs, please see our post titled โDataOps For Business Analytics Teams.โ