Savvy executives maximize the value of every budgeted dollar. Decisions to invest in new tools and methods must be backed up with a strong business case. As data professionals, we know the value and impact of DataOps: streamlining analytics workflows, reducing errors, and improving data operations transparency. Being able to quantify the value and impact helps leadership understand the return on past investments and supports alignment with future enterprise DataOps transformation initiatives.ย Below we discuss three approaches to articulating the return on investment of DataOps.
Resource Redeployment
In a recent Gartner survey (figure 1), data professionals spent 56% of their time on operational execution and only 22% of their time on innovation that delivers value. An effective DataOps strategy can help a team invert this ratio and provide more value to the company.ย
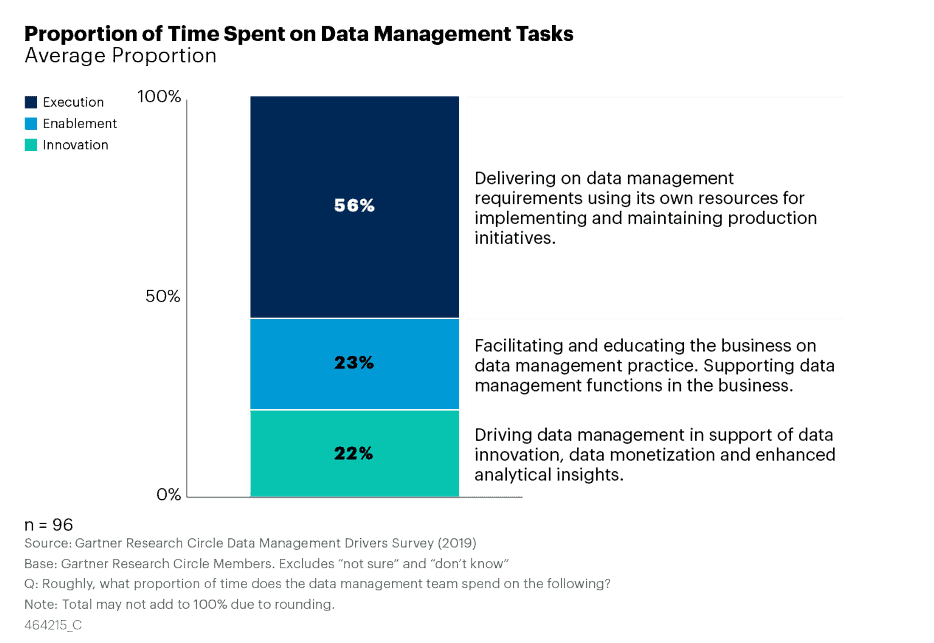
Figure 1: Data professionals spend only 22% of their time on innovation.
Gartner describes the time spent on โoperational executionโ execution as using the data team to implement and maintain production initiatives. A big percentage of the time that data scientists spend on operational effort is consumed servicing data errors.
In teams with mature DataOps practices, including some long-time DataKitchen customers, data professionals have indeed flipped the ratio and spend much less time on nonvalue-added activities.ย Instead, these organizations commit 20% of their time implementing automation and writing tests. As a result, they reduced the time spent on errors and manual processes to nearly zero. This allows the team to spend significantly more time focusing on high-value efforts and meaningful collaborations.ย Good rules of thumb are:
- If youโll perform an operation twice in a year, then automate it.
- If it can be wrong, test it.
Implementing DataOps automation requires about 20% of a data professionalโs time, but it completely eliminates data team participation in operations, saving them 56% of their time; a net savings of 36%. For a team of ten data professionals, this savings is the equivalent of adding more than 3.5 full-time employees to value-added activities. These newly available resources can be redeployed to create more capacity for the companyโs analytics-hungry product teams.
Another way to demonstrate the impact of DataOps on FTEs is by showing the math.
Thirty-six percent of the total time of a ten-person team, based on a full-time employee (FTE) cost of $156,000 amounts to $561,000. This significant sum can be redeployed to higher value-add activities.
Insourcing Through DataOps
Many companies overcome their staffing limitations by outsourcing critical work to third parties. When internal analytics workflows are automated, there is little advantage to outsourcing. With DataOps, the work can often be performed much less expensively through automated orchestrations that are developed and managed in house. Automation can free up both direct and indirect resources. It enables companies to redirect the utilization of their own staff and reduce the dependency on external resources. If your company spends millions on consulting fees and outside contractors, DataOps automation could make a significant contribution to the bottom line. In one real-world example, a DataKitchen customer realized a net savings of $70 million dollars as effort transitioned fully from outside agencies to internal resources.
Cost of Slow Decision Making
What can you do with the resources that are freed up from DataOps automation? One approach applies these resources to business analytics that expedite and improve decision-making.
Analytics agility leads to business agility. When the data team delivers analytics rapidly and accurately, analytics do a better job supporting decision-makers. When an organization can make decisions faster and better, it is able to capture opportunities that it would have otherwise missed or misjudged. With analytics playing a central role in corporate strategy, analytics agility can be a competitive advantage.
In one example, using analytics to understand customers and markets significantly improved product launch success at one DataOps enterprise. With rapidly produced analytics, they were able to improve market segmentation to maximize revenue in the early product lifecycle, boosting lifetime product revenue.
Conclusion
When executives evaluate whether to invest in a DataOps initiative, they need to understand the business benefits. Improved productivity, reduced outsourcing costs, and greater business agility together build a strong business case for DataOps. It may help to start with a mini or pilot project that demonstrates DataOps benefits. Improvement of a key metric may provide the justification that you need to secure investment in a larger DataOps program.

About the Author