A Guide to the Six Types of Data Quality Dashboards
Poor-quality data can derail operations, misguide strategies, and erode the trust of both customers and stakeholders. Data quality dashboards have emerged as indispensable tools, offering a clear window into the health of their data and enabling targeted actionable improvements. However, not all data quality dashboards are created equal. Their design and focus vary significantly depending on an organizationโs unique goals, challenges, and data landscape. This blog delves into the six distinct types of data quality dashboards, examining how each fulfills a specific role in ensuring data excellence. By understanding and selecting the correct type of dashboard, organizations can maximize their influence, streamline efforts, and drive meaningful, sustained improvements in data quality.ย Read more about how your data quality dashboard can be organized by data quality dimension, Critical Data Element (CDE), business goal, data source, and data customer, or it can be based on ticketed workflows.ย
ย 1. Data Quality Dimension-Focused Dashboards
The first type of data quality dashboard emphasizes data quality dimensions. Data Quality Dimension-Focused Dashboards are designed to evaluate data through fundamental quality dimensions, such as completeness, accuracy, timeliness, consistency, and uniqueness. These dimensions provide a best practice grouping for assessing data quality.ย By scoring each dimension individually, these dashboards offer a grouped view of data quality issues, enabling targeted interventions to address gaps.
For example, metrics like the percentage of missing values help measure completeness, while deviations from authoritative sources gauge accuracy. Timeliness can be assessed by tracking the alignment of data updates with business timelines. These metrics are typically visualized through tools such as heatmaps, pie charts, or bar graphs, making it easy for stakeholders to understand compliance levels across different dimensions. A retail company, for instance, might use such a dashboard to monitor the completeness of customer profiles, ensuring marketing campaigns have enough dataโsuch as email addresses and phone numbersโfor effective targeting.ย
Benefits and Challenges of The Data Quality Dimension Approach
Data quality dimensions are often heralded as foundational principles for evaluating and improving data quality. While these dimensions provide a โscientificโ way to assess data, they can be overly general when applied to real-world data quality problems. While conceptually appealing, this generality can limit their practical utility in driving meaningful improvements in data quality.ย
The issue lies in the abstraction of these dimensions. For example, a metric like โcompletenessโ is essential to data quality, but defining what โcompleteโ means in a specific context can vary widely. Is completeness about filling every field in a record, or is it about having the fields critical to a particular business process? Similarly, โaccuracyโ often assumes the existence of an authoritative source for validation, which may not always be available or practical to implement. Without contextual specificity, these dimensions risk becoming check-the-box exercises rather than actionable frameworks that help organizations identify and address the root causes of data quality issues.
โThe DAMA Data Quality Dimension dashboards are crap.ย They do nothing to motivate people to make improvements to their data.โย โ Senior Data Quality Person, Financial Services, DataKitchen Market Research 2024
Moreover, relying solely on DAMA dimensions tends to produce dashboards disconnected from an organization’s or its stakeholders’ unique needs. A dashboard filled with generic scores for completeness or consistency may look polished but needs to resonate with the teams responsible for acting on the insights. For example, a business executive might struggle to connect a โ70% consistency scoreโ with the operational goals they are trying to achieve. Similarly, data teams might struggle to determine actionable steps if the metrics do not highlight specific datasets, systems, or processes contributing to poor data quality.
While the DAMA dimensions provide a starting point, the key to impactful dashboards is translating these principles into concrete, tailored insights that directly address the organization’s challenges and objectives. By narrowing the scope and aligning metrics with actionable outcomes, organizations can ensure that their dashboards assess data quality and actively contribute to its enhancement.
2. Critical Data Element (CDE) Data Quality Dashboards
Critical Data Element (CDE) dashboards focus on essential data elements crucial for business operations or regulatory compliance. These dashboards prioritize high-impact fields such as financial values, key identifiers like customer IDs, or data required to meet regulatory standards. By narrowing their scope to these critical elements, CDE dashboards enable organizations to allocate resources efficiently and address the data quality with the most significant impact. For instance, a bank might use a CDE dashboard to monitor the accuracy of customer credit scores, which are vital for regulatory compliance and effective risk modeling. Another example is an analytic team that wants to focus on data that goes into the weekly report for the executive team.ย This targeted approach ensures that limited resources are directed toward improving the data elements that matter most to the organization.
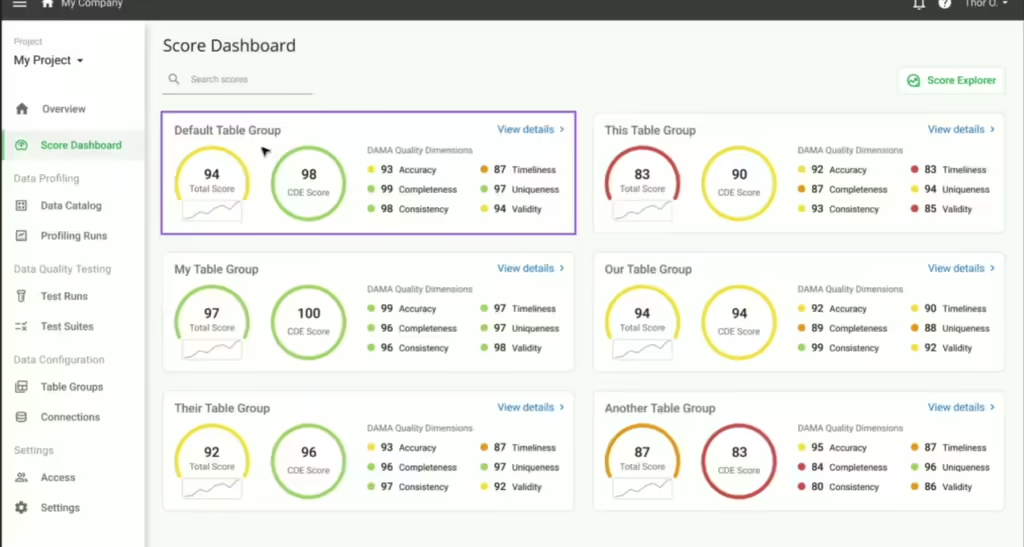
An example of a data quality dashboard with CDEs from DataKitchen’s DataOps Data Quality TestGen Open Source Software.
Benefits and Challenges of The CDE Approach
Critical Data Element (CDE)–based data quality dashboards are highly effective in regulated industries such as finance, healthcare, and utilities, where organizations are mandated to monitor and report on the quality of specific data elements. CDEs are often clearly defined by regulatory bodies or industry standards in these industries. For example, a financial institution may need to ensure the accuracy and completeness of loan application data to comply with anti-money laundering (AML) regulations.. The specificity of these mandates makes it easier to identify CDEs and prioritize resources to ensure their quality. CDE-based dashboards in such contexts serve as powerful tools for compliance, risk mitigation, and operational oversight, providing clarity and focus on what truly matters.
โWe have had amazing Data Quality Improvement, aligning everyone to improve data based on CDEs.ย We have corporate goals on CDE data quality.ย Why?ย Because we MUST do them for compliance reporting to the government.ย We focus our Data Quality reporting on CDEs.โย โ Senior Data Quality Leader, Large US Bank Services, DataKitchen Market Research 2024
However, identifying and gaining consensus on what constitutes a CDE is a significant challenge in industries without strict regulatory frameworks. Unlike regulated industries, where the importance of specific data elements is externally prescribed, non-regulated industries must internally determine which data elements are critical to their success. This process often involves aligning diverse stakeholdersโfrom business leaders to data engineersโwho may have varying priorities and definitions of โcritical.โ For instance, a marketing team might prioritize customer segmentation data, while operations teams focus on inventory data. The lack of predefined criteria can lead to prolonged debates, misalignment, and inconsistent interpretations of what should be considered a CDE.
Another challenge in non-regulated industries is the dynamic nature of business priorities. CDEs may shift as market conditions, organizational goals, or technologies evolve. A retail organization, for example, might initially consider transaction data as critical, only to pivot toward prioritizing clickstream data for e-commerce analytics. This fluidity requires an iterative approach to defining and managing CDEs, which can be resource-intensive and complicated to operationalize within a dashboard framework.
Despite these challenges, CDE-based dashboards remain valuable in non-regulated industries if implemented thoughtfully. Organizations can address these hurdles by establishing transparent governance processes for identifying CDEs, leveraging cross-functional collaboration to ensure alignment, and periodically revisiting and revising the CDE list to reflect changing priorities. While it requires effort and coordination, the result is a targeted approach to data quality that delivers tangible business value, even in industries where regulations donโt dictate the terms.
3. Business Goal-Focused Data Quality Dashboards
Business goal-focused data quality dashboards link data quality metrics directly to specific organizational objectives, such as quarterly revenue goals, yearly cost savings targets, or specific revenue-generating marketing and sales programs. These dashboards highlight how data quality influences broader goals, such as customer retention, revenue growth, or regulatory compliance. For leadership teams, this type of dashboard serves as a critical tool to understand the operational and financial implications of data quality issues and to justify investments in remediation efforts. For example, an e-commerce company might use a business goal-focused dashboard to monitor how incomplete customer delivery information affects order fulfillment rates and timing, a crucial metric for customer satisfaction. By tying data quality improvements directly to business outcomes, these dashboards make the case for prioritizing data quality as a strategic initiative.
Benefits and Challenges of The Business Goal-Focused Data Quality Approach
Business goal-focused data quality dashboards offer a way to connect technical data quality efforts with broader organizational objectives. By focusing on outcomes that matter to business stakeholders, these dashboards make data quality improvements more relevant and impactful, fostering cross-functional collaboration between technical teams and business units. This alignment also helps secure executive buy-in and funding for data initiatives by demonstrating the tangible value of addressing data quality issues.
Another significant advantage is the ability of these dashboards to provide ROI-driven insights. By highlighting the financial or operational impact of poor-quality data, business goal-focused dashboards allow organizations to prioritize remediation efforts that deliver the most significant value. For example, identifying how incomplete customer delivery information affects fulfillment rates can help an e-commerce company streamline operations, reduce costs, and enhance customer satisfaction.ย
โHow do you get the business to take action?ย By definition, they are inputting data that meets their data quality needs.ย With all their other problems, why should they care about data quality when providing data to other teams? ย The only way to motivate them is to pick data elements directly affecting their quarterly business goals.ย They care about that.โย โ Data Quality Consultant, DataKitchen Market Research 2024
Despite their advantages, business goal-focused dashboards come with notable challenges. One of the most significant is the difficulty of defining and maintaining the alignment between data quality metrics and business goals. Business objectives often evolve due to market dynamics, organizational restructuring, or technological changes, requiring dashboards to be continuously updated to stay relevant. This iterative process demands time, effort, and team collaboration, which can strain resources, especially in organizations with limited data governance capabilities.
4. Data Source-Focused Data Quality Dashboards
A data source-focused dashboard assesses data quality by analyzing its origin, enabling organizations to identify sources that consistently provide low-quality data and take corrective actions. This approach allows enterprises to hold data suppliers accountable or optimize their ingestion processes to ensure higher data integrity. By tracking source-level metrics such as error rates, duplication, and timeliness, these dashboards pinpoint the suppliers or systems responsible for data quality failures, fostering accountability and improvement. This is particularly valuable for organizations managing data from multiple external providers or systems, such as suppliers or third-party APIs. For example, a logistics company might use a source-focused dashboard to monitor the accuracy and timeliness of shipping data from various courier partners, ensuring reliable tracking information for customers and maintaining service quality.
Benefits and Challenges of Data Source-Focused Data Quality Dashboard Approach
Data source-focused dashboards assign accountability for data quality. Organizations can take targeted action by identifying which suppliers, systems, or processes are contributing to poor-quality data, whether that means renegotiating contracts with external vendors, improving internal workflows, or implementing better validation processes at the point of ingestion. This accountability encourages data providers to prioritize quality.
Another significant benefit is these dashboards’ actionable insights for optimizing data ingestion processes. Organizations can track each source’s error rates and timeliness. This enables them to refine pipelines and ensure data flows into the organization more accurately and reliably. For example, a logistics company might use these dashboards to monitor how courier partners provide shipping data, identify lagging contributors, and take steps to ensure timely updates.ย
โOur data suppliers donโt know that we exist, donโt care about quality, and will make arbitrary changes to our feeds at any time. ย Itโs up to us to deal with it. ย We have no control over their data qualityโย โ Data Engineer, Pharmaceuticals, DataKitchen Market Research 2024
Despite their advantages, data source-focused dashboards have notable challenges. One challenge lies in gaining cooperation from external data providers. While organizations can monitor and report on data quality issues, enforcing improvements depends on the willingness and capability of suppliers to address them. They often do not care.ย How do you motivate them to make a change? These dashboards can become less effective when multiple sources contribute to a single dataset, making it difficult to pinpoint the root cause of data quality issues. For example, if data from various suppliers is aggregated into a central system, determining whether errors originated with a specific supplier or during the integration process requires a root cause analysis.ย
5. Data Consumer-Focused Data Quality Dashboards
A data consumer-focused dashboard is designed to meet the specific needs of individuals and teams who rely on data to perform their roles, such as data scientists, analysts, or business intelligence professionals. These dashboards prioritize the quality and reliability of datasets and features critical to analytics models, reports, or decision-making tools, ensuring they are fit for purpose. They are often customized to address the unique requirements of different user personas, whether for predictive model inputs or operational reporting. For instance, a healthcare company might use such a dashboard to monitor the accuracy and consistency of patient demographic data utilized in predictive models for patient readmittance models. By catering directly to the needs of data consumers, these dashboards help their data customer use their influence to make changes to improve data quality.
Benefits and Challenges of Data Consumer-Focused Data Quality Dashboard Approach
One of the primary benefits of this approach is its emphasis on ensuring that the data used by analysts, data scientists, and other consumers is reliable, accurate, and error-free. Tracking the quality of specific datasets or features critical to their projects can give the project owner leverage to improve data quality. Another key advantage is the customization these dashboards provide, tailoring metrics and views to the needs of individual roles or teams. Different data consumers have unique requirements: a business intelligence analyst may need dashboards that track the completeness of reporting data. At the same time, a data scientist might need to focus on model input features such as timeliness or uniqueness. By aligning data quality metrics with the specific needs of end-users, these dashboards foster efficiency and confidence in analytics processes.ย
โOur data scientists are forever complaining about the quality of data.ย They have high-value predictions in production.ย They are motivated to drive improvements to data. ย We need to focus our data quality reporting on the data inputs to their models, specifically. โ Data Quality Consultant, DataKitchen Market Research 2024
While highly effective, data consumer-focused dashboards present several challenges. One significant hurdle is defining and managing the diverse requirements of different user groups within an organization. Each team or individual may have distinct data needs, leading to a complex and time-intensive process of creating and maintaining customized dashboards. Balancing these needs while ensuring the scalability and manageability of the dashboard system is critical.
6. The Data Quality Ticket Focused Dashboard
Using tickets to drive data quality improvements is another approach to managing and resolving data issues. Tickets are change requests that break down data quality challenges into specific, detailed tasks targeting individual data elements or processes. Each ticket represents a well-defined issueโsuch as fixing missing values, correcting data inaccuracies, or standardizing inconsistent formatsโmaking it easier for data engineering teams to prioritize, assign, and resolve problems systematically. This approach ensures that data quality initiatives are not abstract or overwhelming but are actionable, trackable, and aligned with organizational goals.
โWe donโt use a dashboard per se; we use workflow. ย It is about the number of tickets created and fixed during a particular period.ย We have specific goals around tickets, and that works for us.โ โ Data Quality Leader, Regional Bank, DataKitchen Market Research 2024
In this context, the data quality dashboard shifts its focus from traditional quality metrics to operational progress, visualizing the state of the ticketing process. The dashboard provides two critical views: the count of tickets completed over time, which highlights progress and the teamโs capacity to address issues, and a prioritized backlog of pending tickets, ensuring that high-impact problems are addressed first. By tracking ticket completion trends, organizations can measure the efficiency of their data quality efforts and identify bottlenecks in the remediation process. This ticket-driven model fosters accountability and aligns with agile principles, enabling continuous iteration and improvement in data quality over time.
Why You Need a Multi-Dashboard Approach: Maximizing Your Influence
Adopting a multi-dashboard approach to data quality is essential for organizations aiming to understand their data landscape comprehensively. Each type of dashboard offers a unique lens through which to view and address data quality issues, and combining these perspectives allows for a more nuanced and effective strategy. By leveraging multiple dashboards, organizations can ensure that no aspect of data quality is overlooked, that priorities are aligned with business goals, and that all stakeholders have access to the data they need to succeed.
Dimension-focused dashboards are foundational in ensuring that every critical aspect of data qualityโcompleteness, accuracy, timeliness, consistency, and uniquenessโis monitored and addressed. These dashboards provide a holistic overview of data quality, enabling organizations to identify and remediate deficiencies systematically. However, this broad approach can be complemented by Critical Data Element (CDE) dashboards, which prioritize the data elements that are most crucial for operational efficiency or regulatory compliance. These dashboards balance addressing general quality metrics and focusing on high-priority data elements.
Business goal-focused dashboards add another layer of alignment by tying data quality efforts directly to organizational objectives. These dashboards ensure that data quality initiatives are not just technical exercises but are integral to achieving key business outcomes such as customer retention, revenue growth, or compliance. At the same time, data source-focused dashboards bring accountability into the equation by identifying where data quality issues originate, whether from internal systems or external suppliers, and streamlining processes to mitigate risks at their source.

An example of a data quality dashboard drill down to specific, actionable issues from DataKitchen’s DataOps Data Quality TestGen Open Source Software.
Finally, data consumer-focused dashboards ensure that end-usersโdata scientists, analysts, and business teamsโcan access reliable and fit-for-purpose data for their needs. Organizations can enhance decision-making, reduce inefficiencies, and build trust in their data ecosystem by catering to these distinct roles. These six dashboards create a comprehensive framework that empowers organizations to address data quality while targeting specific pain points.
Best Practices for Building Effective Data Quality Dashboards
The first step is to define clear objectives for each dashboard. Understanding its specific purposeโwhether to monitor compliance, support operational goals, or provide data consumers with reliable insightsโhelps tailor the metrics to align with organizational priorities. A well-defined objective ensures that dashboards remain focused and deliver value to stakeholders without overwhelming them with irrelevant or extraneous data.
Automation is another critical element in maximizing the effectiveness of data quality dashboards. Fast updates ensure that dashboards remain responsive to the latest data conditions, reducing the need for manual intervention and improving decision-making speed. Automation also enhances scalability, making maintaining multiple dashboards across an organization easier without excessive overhead. By leveraging modern tools and frameworks like DataKitchenโs DataOps Data Quality TestGen, teams can ensure their dashboards remain dynamic and relevant, even as data sources and business requirements change.
Accessibility is critical to ensuring that dashboards are effective for technical and non-technical stakeholders. Dashboards should be intuitive, with user-friendly visualizations and interfaces catering to end-users’ diverse needs. Clear, actionable metrics and insights allow executives, data scientists, and operations teams to understand and act on data quality issues without requiring extensive technical expertise. Dashboards must be paired with processes to act on their insights to influence data quality outcomes. Dashboards alone are diagnostic toolsโthey highlight problems but cannot solve them. Organizations should integrate their dashboards with fast, actionable, motivating. โHere fix thisโ ticket creation and workflows that enable rapid remediation of issues. This action-oriented approach ensures that data quality issues identified by dashboards are fixed.
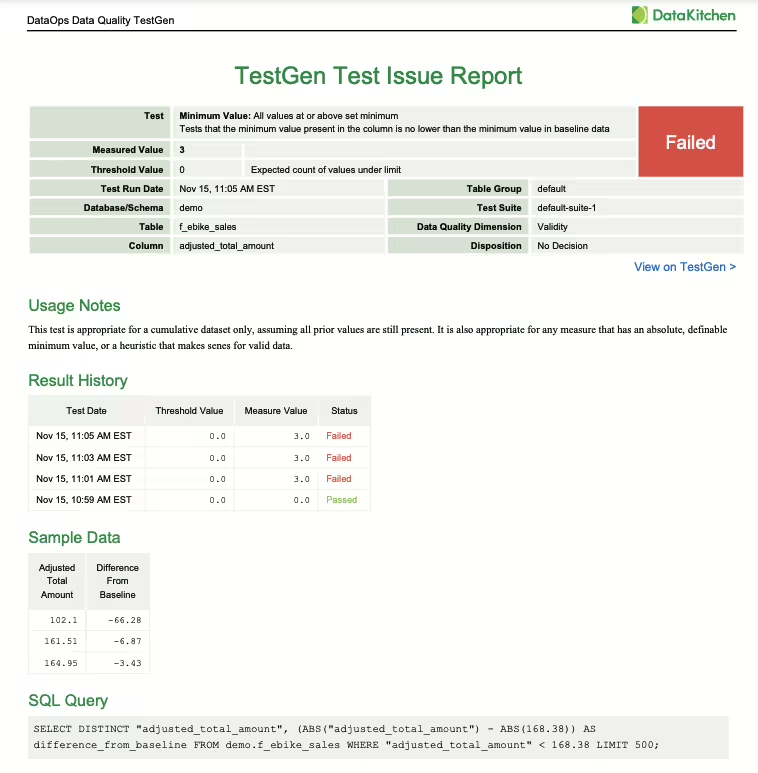
A Data Quality Issue Report from DataKitchen’s DataOps Data Quality TestGen Open Source Software
Iteration and continuous improvement are essential to the long-term success of data quality dashboards. As organizational needs evolve, so must the dashboards and the metrics they track. A DataOps approach to data quality is needed, emphasizing collaboration, automation, and iterative development. DataOps enables teams to innovate on dashboards and metrics, ensuring they remain aligned with changing priorities and drive sustained improvements. By iterating and innovating, organizations can maximize their influence on stakeholders and foster a culture of continuous data quality enhancement.
Conclusion
Data quality dashboards are indispensable tools for managing and improving data quality. By leveraging the six types of dashboardsโdimension-focused, CDE, business goal-focused, data source-focused, data consumer-focused, and task list โorganizations can take a strategic and targeted approach to address their unique data quality challenges. The goal is to achieve agile data quality at scale: automate data quality tests, make informed trade-offs, iterate quickly to maximize your influence, and deliver actionable improvements to those who can make changeโfast and focused. With the right dashboards, your organization can move confidently toward its data-driven goals and ensure that decisions are built on a solid foundation of high-quality data.ย And you, as a data quality leader, can gain influence and success in your organization!
ย