What is data lineage?
Data lineage traces data’s origin, history, and movement through various processing, storage, and analysis stages. It is used to understand the provenance of data and how it is transformed and to identify potential errors or issues. Data lineage can also be used for compliance, auditing, and data governance purposes. Data lineage has a long history, starting as a tool for compliance and auditing in mainframe systems, evolving to address the challenges of understanding the origin, history, and movement of data across multiple systems and processes, and becoming a critical aspect of data governance, compliance, and data management in recent years, with the growing complexity of data and the increasing importance of data privacy and security. Data lineage and a data catalog are better together because they provide a more complete and accurate view of the data.
What about DataOps Observability? How does it compare?
A five-on-five comparison of data lineage vs. DataOps Observability
Five on data lineage:
- Data lineage traces data’s origin, history, and movement through various processing, storage, and analysis stages.
- Data lineage helps to understand the provenance of data and how it is transformed and identify any potential errors or issues.
- Data lineage is important for data governance, compliance, and auditing purposes.
- Data lineage can be used to create a data dictionary or data catalog, which can be used to understand the data better.
- Data lineage is typically stored in separate systems from the data itself and can be difficult to keep up-to-date.
Five on DataOps Observability:
- DataOps Observability is the ability to understand the state and behavior of data and the software and hardware that carries and transforms it as it flows through systems. It allows organizations to see how data is being used, where it is coming from, its quality, and how it is being transformed.
- DataOps Observability includes monitoring and testing the data pipeline, data quality, data testing, and alerting.
- Data testing is an essential aspect of DataOps Observability; it helps to ensure that data is accurate, complete, and consistent with its specifications, documentation, and end-user requirements.
- Data testing can be done through various methods, such as data profiling, Statistical Process Control, and quality checks.
- DataOps Observability is crucial for identifying and addressing issues with the data pipeline, including quality and timeliness, improving data governance, end-user satisfaction, and ensuring compliance with regulations.
Your house is on fire: look at blueprints or listen to smoke alarms? What is missing in data lineage?
Data lineage answers the question, “Where is this data coming from, and where is it going?” It is a way to describe the data assets in an organization. It is a description used to help data users understand where data came from and, with a data catalog, the content of specific data tables or files. Data Lineage does not answer other key questions, however. For example, data lineage cannot answer questions like: “Can I trust this data used in this specific pipeline in this way?” “And if not, what happened during the data journey that caused the problem?” “Has this data been updated with the most recent files?”
Understanding a complete data journey and building a system to monitor that complex series of steps is an active, action-oriented way of improving the results you deliver to your customers. DataOps Observability solutions create and describe Data Journeys representing this run-time lineage.
Think of it this way: if your house is on fire, you don’t want to go to town hall and get the blueprints of your home to understand better how the fire could spread. You want smoke detectors in every room so you can be alerted quickly to avoid damage. Data Lineage is the blueprint of the house; DataOps Observability is the set of fire detectors sending you signals in real time. Ideally, you want both run time and data lineage to provide the complete picture.
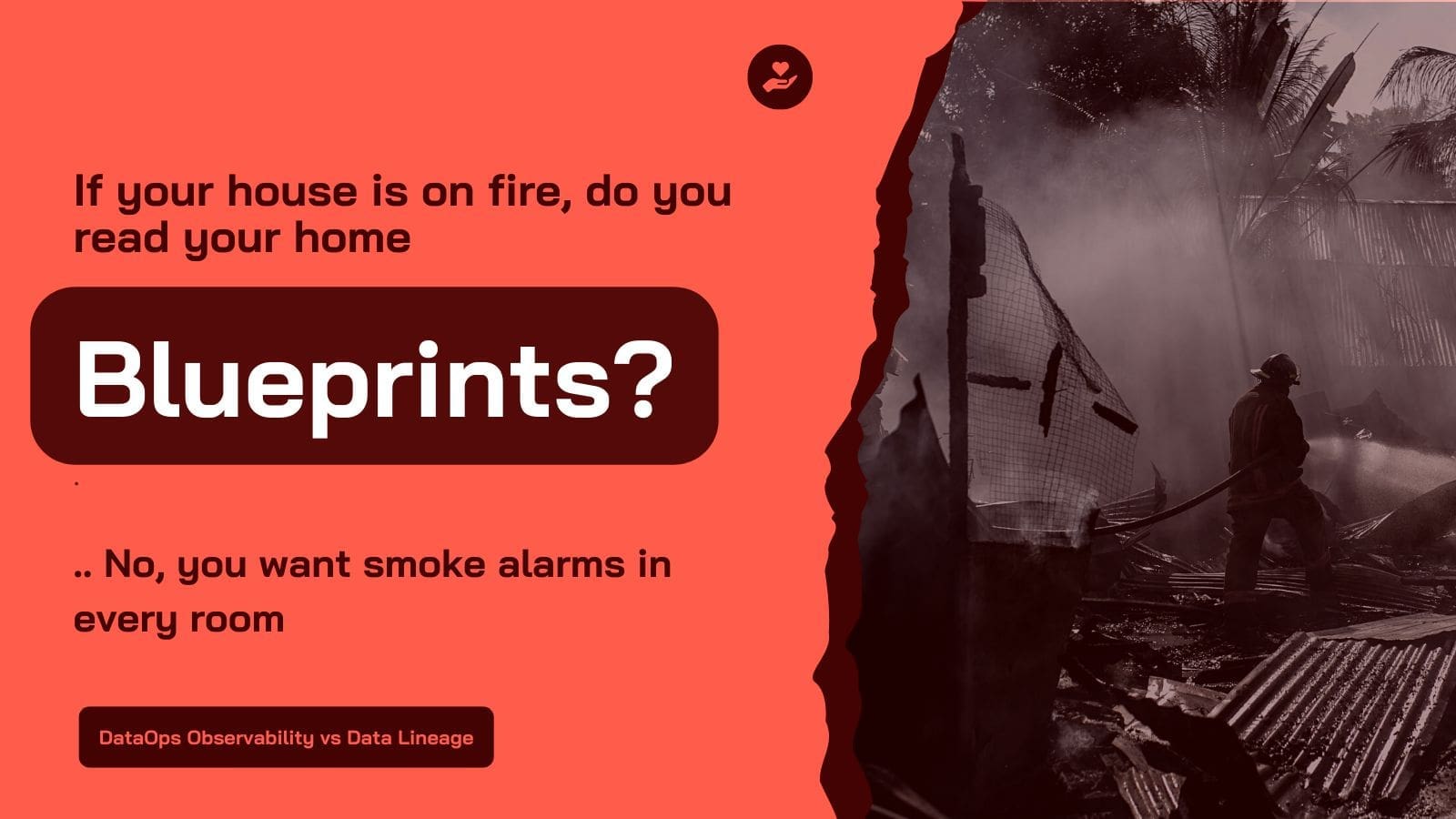
Data Lineage’s trust gap.
While data lineage can provide a clear understanding of the origin, history, and movement of data, it may not always fully capture the impact of a data error. Data lineage primarily focuses on tracking the movement of data and how it is transformed. Still, it may not always provide a complete picture of how data is used or how it affects other systems or processes.
Data errors can have a wide range of impacts depending on the nature of the error and how it propagates through the data pipeline. This impact can be hard to predict and understand without additional context. For example, a data error may only be apparent when combined with other data or used in a specific analysis or report. Additionally, data lineage may not capture the impact of data errors on downstream systems or processes. For example, if an error in the data causes a downstream system to fail, data lineage may not capture this information.
In summary, data lineage can provide a clear understanding of the origin, history, and movement of data, but it may not always fully capture the impact of a data error. It is important to have additional tools and processes in place to understand the impact of data errors and to minimize their effect on the data pipeline and downstream systems.
Data lineage vs. the run time operations on data
Runtime operations, such as those captured and monitored by DataOps Observability solutions, refer to the actions performed on data while it is being processed. These operations can include data movement, validation, cleaning, transformation, aggregation, analysis, and more. They are performed on the data during production runtime when it is actively processed.
Data lineage can provide information about how data is transformed and moved, but it does not provide information about the specific runtime operations performed on the data. Conversely, runtime operations can impact the data but not provide information about its history, movement, and origin.
Data lineage and runtime operations on data are related but distinct concepts. Data lineage provides information about the origin, history, and movement of data, while runtime operations provide information about the actions performed on data while it is being processed. Both concepts are important for understanding and managing data effectively.
Data observability and data lineage are complementary concepts.
Data lineage refers to tracing data’s origin, history, and movement through various processing, storage, and analysis stages. It is used to understand the provenance of data and how it is transformed and to identify any potential errors or issues.
On the other hand, DataOps Observability refers to understanding the state and behavior of data as it flows through systems. It allows organizations to see how data is being used, where it is coming from, and how it is being transformed. This includes other information such as data quality metrics, processing steps, timing, data test results, and more.
As such, data lineage and DataOps Observability have their own separate capabilities and goals, which, when used together, deliver higher-quality systems and products.
Trust comes from verification first, then root cause analysis
Trust in data, which is critical for the successful use of data, comes from verification first, then understanding the root cause of any issues second. Verification is checking that data is accurate, complete, and consistent with its specifications or documentation. This includes checking for errors, inconsistencies, or missing values and can be done through various methods such as data profiling, data validation, and data quality assessments.
Once the data has been verified, it is important to understand the root cause of any identified issues. This includes identifying where the data came from, how it was collected, and how it was transformed before it was stored in the database. Understanding the root cause of issues can help prevent them from happening again in the future and improve data governance. Data Lineage can be one of many helpful tools in diagnosing root-cause errors.
Data lineage is what’s in your database – which is not everything.
Data lineage primarily focuses on tracking the movement and transformation of data within the database or data storage systems. It does not capture the full details of all the steps and tools used to process and analyze the data before storing it in the database. DataOps Observability handles that.
Data lineage in a database typically includes information about where the data came from, when it was created or last modified, and who created or modified it. However, it does not typically include information about the tools or processes used to collect, clean, or transform the data before it was stored in the database.
To capture a more complete picture of the data’s journey, it is important to have a data pipeline management and monitoring system that can track the data flow and all the steps and tools it went through before it reaches the database. This additional information can help to understand the data better, troubleshoot issues, and improve data governance. To capture a more complete picture of the data’s journey, it is important to have a DataOps Observability system in place.
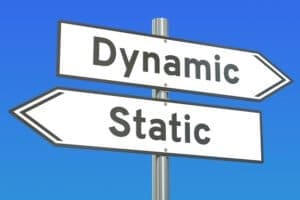
Data lineage is static and often lags by weeks or months.
Data lineage is often considered static because it is typically based on snapshots of data and metadata taken at a specific time. This means that the data lineage information may not reflect the current state of the data or the most recent changes that have been made. Additionally, data lineage information is often stored in separate systems from the data itself, making it difficult to keep it up to date and in sync with the data. These factors can contribute to outdated data lineage lagging by weeks or months. This can make it difficult to understand the current state of the data and how it is being used, which can be problematic for data governance, compliance, and auditing purposes. Additionally, in situations where data is frequently updated or transformed, this lag in data lineage can make it difficult to identify issues or errors with the data. However, a DataOps Observability capability, by monitoring data processes in real-time, can create and feed a lineage system with the latest details, ensuring the information is up to date.
Data lineage fails at impact analysis.
You are in bed, smell smoke, touch the door nob, and it’s hot. Is it time to get the blueprints of your apartment out to check which room will burn next? Like an apartment blueprint, Data lineage provides a written document that is only marginally useful during a crisis. This is especially true in the case of the one-to-many, producer-to-consumer relationships we have on our data architecture. Which report tab is wrong? Which production job filled that report? When did it last run? Did it fail? Are problems with data tests? These questions are much more salient and actionable than pulling out the dusty old data lineage blueprint. Data Observability trumps lineage in a production crisis.
But what about during development? Doesn’t lineage help me tell the impact of my code change? Yes, it does – to a point. Data lineage does not provide detailed regression, functional, or performance test results. Testing data and analytic systems require a development system with accurate test data, tools, and relevant tool code. All of those things come together with an overlay of automated tests. Only then can you tell the true impact of a column name change on the data transformations, the models, and the visualization you give to your customers. No software engineer trusts an abstract metamodel of their code to prove that it works in production without running their software code through a series of rigorous automated tests before it gets to production. We must do the same as data analytic teams. DataOps Observability enables this.
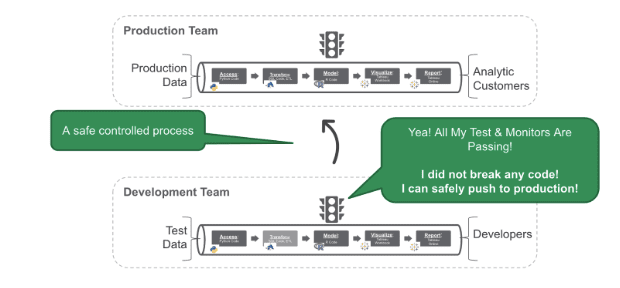
Code and tools can cause production problems — data lineage does not help.
One view of data and analytic systems is that it is a grouping of tools, driven by code, acting in a specified order upon data. Data lineage alone can not fully capture the issues caused by code and tools acting upon data. Data lineage primarily focuses on tracking the movement and transformation of data, but it does not provide information about the code and tools used to process and analyze the data.
Code and tools can introduce errors or issues with the data, such as bugs, incorrect logic, or unexpected behavior, that data lineage may not capture. Additionally, if the code or tools are not properly tested, monitored, or maintained, they may introduce issues that are not immediately apparent over time.
To address these issues, it is important to have additional processes and tools in place, such as code review, testing, and monitoring, to ensure that the code and tools are functioning correctly and to detect any issues as early as possible. Data lineage can still provide valuable information about the data. Still, it should be used with DataOps Observability tools to understand and address issues caused by code and tools fully.
Data lineage does not directly improve data quality.
Though valuable, Data Quality scores are largely static. They measure data sets at a point in time. DataOps Observability is dynamic; it is the testing of data, integrated data, and tools acting upon data — as it is processed — that produces details on data timeliness, flow rates, and errors. A financial analogy: Data Quality is your Balance Sheet, and Data Observability is your Cash Flow Statement – any successful business requires both. Crafting your data observations into a singular Data Journey that integrates all tools, tech, data, and results in a single view .. that is DataOps Observability.
Data reality vs. the data lineage specification.
In reality, data often differs from its specifications or stated lineage due to a variety of factors. One common reason is that data is often collected and entered into systems by humans, and human error can lead to inaccuracies or inconsistencies. Additionally, data may be transformed or cleaned during processing, resulting in changes to the data that are not reflected in the original lineage or documentation. Furthermore, data may not be kept up to date or stored in multiple locations, making it difficult to reconcile the data with its original lineage.
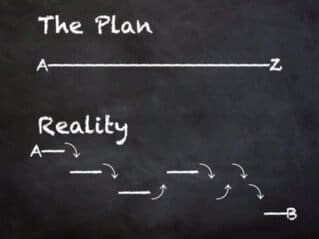
Another reason for the discrepancy between reality and specifications is that data is often collected from various sources, which may not have the same accuracy or completeness. Some data may also be missing or incomplete, leading to missing values, outliers, or other issues. Furthermore, data may be updated over time, but the original specifications may not be updated accordingly. In addition, the context of data usage may change over time, leading to the data being used in ways that were not originally intended, leading to misinterpretation and errors.
Reality and specifications for data often differ due to various factors such as human error, the transformation of data during processing, lack of up-to-date documentation, data collected from various sources, data’s context change over time, and more. This can make it difficult to understand the data’s current, correct lineage and use it effectively.
One way to ensure that data meets the specifications and, more importantly, the business requirements is to track and monitor all data processes with a DataOps Observability solution.
Managing data team stress: A brain scan vs. cognitive behavior therapy
In a recent DataKitchen/data.world survey, 97% of data engineers report experiencing burnout in their day-to-day jobs. 70% say they will likely leave their current company for another data engineering job in the next 12 months. 79% have considered leaving the industry entirely.
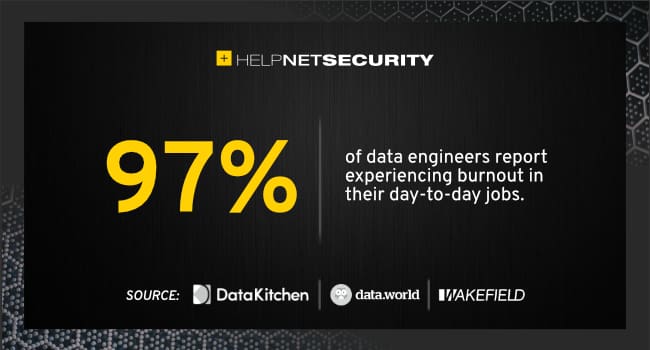
What is the best way to solve this stress? Let’s try another analogy: a brain scan vs. cognitive behavior therapy. Brain scans, such as functional magnetic resonance imaging (fMRI) or positron emission tomography (PET) scans, create detailed brain images to understand how it functions. While brain scans can provide valuable information about brain activity, they are not typically used as a standalone treatment for anxiety. Cognitive-behavioral therapy (CBT) is a type of talk therapy that focuses on changing how a person thinks and behaves to reduce anxiety. It involves identifying and changing negative thought patterns and behaviors contributing to anxiety. CBT is an effective treatment for anxiety and is recommended by the National Institute of Mental Health (NIMH) as a first-line treatment.
Data Lineage is like a brain scan of your data. In contrast, DataOps Observability uses a series of data tests and monitors to find those ‘negative thought patterns’ in your data and notify you immediately. Like CBT, DataOps Observability effectively treats data team anxiety, while data lineage is used to create detailed images of the state of your data tables to understand how it functions.
Data lineage can provide valuable information, but it fails as a standalone treatment for data team anxiety. However, it can be used with other treatments to understand the underlying mechanisms of data anxiety and to help tailor treatment!
Conclusion
In the 1990’s RomCom Jerry Maguire, the main characters have this iconic exchange:
Jerry Maguire: [babbling and struggling] I love you. You… you complete me. And I just…
Dorothy: Shut up,
[pause]
Dorothy: Just shut up.
[Pause]
Dorothy: You had me at “hello.” You had me at “hello.”
We’ve tried to show the benefits of a long-lasting relationship between DataOps Observability and data lineage. Both can help give a complete view of your data and operations. Together, both tools help deliver more trusted insight to your customer.
Now, go ahead and “Show Me The Money” (with your data)!
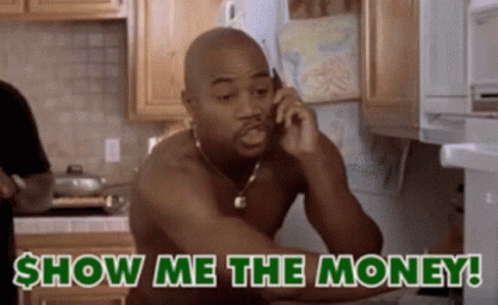