On 20 July 2023, Gartner released the article “Innovation Insight: Data Observability Enables Proactive Data Quality” by Melody Chien.
In the article, Melody Chien notes that Data Observability is a practice that extends beyond traditional monitoring and detection, providing robust, integrated visibility over data and data landscapes. It alerts data and analytics leaders to issues with their data before they multiply. The need for better observability over data quality and data pipelines is increasing due to distributed data landscapes, datasets diversity, and high data quality requirements. Traditional monitoring tools need to be more comprehensive to address new issues not previously understood or detected. Data Observability technology learns what to monitor and provides insights into unforeseen exceptions. However, the market for Data Observability is fragmented and lacks a standard accepted definition, leading to confusion and tool adoption issues.
She sees Data Observability as an emerging technology in data engineering and management. It fills the gap for organizations needing better visibility of data health and data pipelines across distributed landscapes beyond traditional network and infrastructure monitoring. Data Observability is the ability to holistically understand the state and health of an organization’s data, data pipelines, data landscapes, data infrastructures, and financial governance. This is accomplished by continuously monitoring, tracking, alerting, analyzing, and troubleshooting problems to reduce and prevent data errors or downtime.
In her view, Data Observability provides five critical observations: observing data content, observing data flow and pipeline, observing infrastructure and compute, observing users, usage, and utilization, and observing financial allocation. Many tools cover some of these five observations individually. Still, these tools focus on certain areas and do not provide holistic and end-to-end data landscape and pipeline visibility. Data Observability platforms fill the gaps and consolidate all available information to create visibility. Most vendors focus on the first two observations — observing data content and data flow.
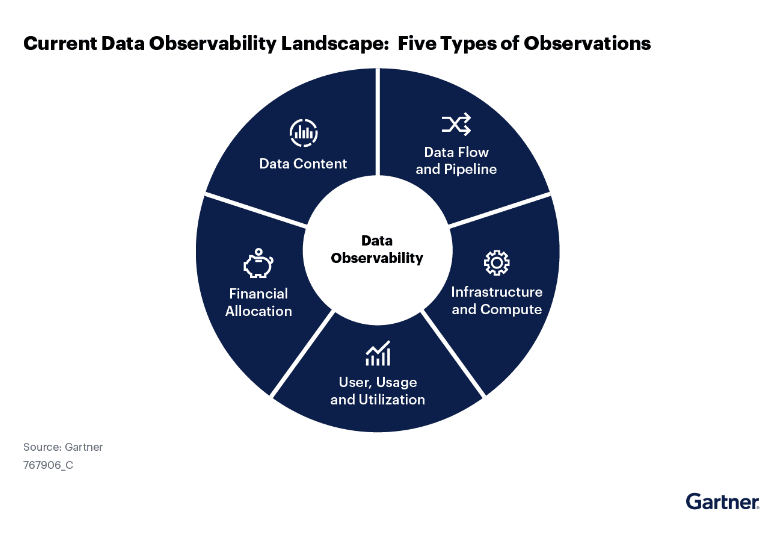
Gartner Types of Data Observations
The main difference, in Chien’s view, is that Data Observability provides greater coverage in monitoring. It is more powerful than simple detection and monitoring. It also performs root cause analysis and provides recommendations to solve problems. Detection and monitoring features are mandated, while root cause analysis and recommendations are considered an important market differentiator among vendors. Data Observability leverages five critical technologies to create a data awareness AI engine: data profiling, active metadata analysis, machine learning, data monitoring, and data lineage.
Finally, the article states that Data Observability can bring tremendous benefits and time savings by helping IT and business teams be more proactive in their data management and engineering activities. It can improve data quality, mitigate risk and adhere to governance requirements, detect unexpected or unknown events, reduce time to investigate and solve issues, improve budget planning and cost control, and increase efficiency and productivity.
However, there are potential risks and challenges in adopting Data Observability. There needs to be a standard definition of what constitutes a Data Observability solution, leading to confusion in the market. The current vendor landscapes are fragmented based on coverage areas and data environments supported. Most Data Observability tools support only modern data stacks, limiting their application in large enterprise environments. Data Observability doesn’t deliver data or contain data remediation capabilities. Therefore, it does not replace data quality solutions.
The Missing Piece Observing ‘Data in Use’ – DataKitchen’s Perspective
From DataKitchen’s perspective, Comparing Data Observability to an end-to-end Data Journey concept is crucial for effective data management. Let’s start with a scenario. You are in bed, smell smoke, touch the door nob, and it’s hot. Is it time to get the blueprints of your apartment out to check which room will burn next? Like an apartment blueprint, Data lineage provides a written document that is only marginally useful during a crisis. This is especially true regarding our one-to-many, producer-to-consumer relationships on our data architecture. Which report tab is wrong? Which production job filled that report? When did it last run? Did it fail? Are problems with data tests? These questions are much more salient and actionable than pulling out the dusty old data lineage blueprint. Data Journeys trumps data lineage in a production crisis. Why is this the case?
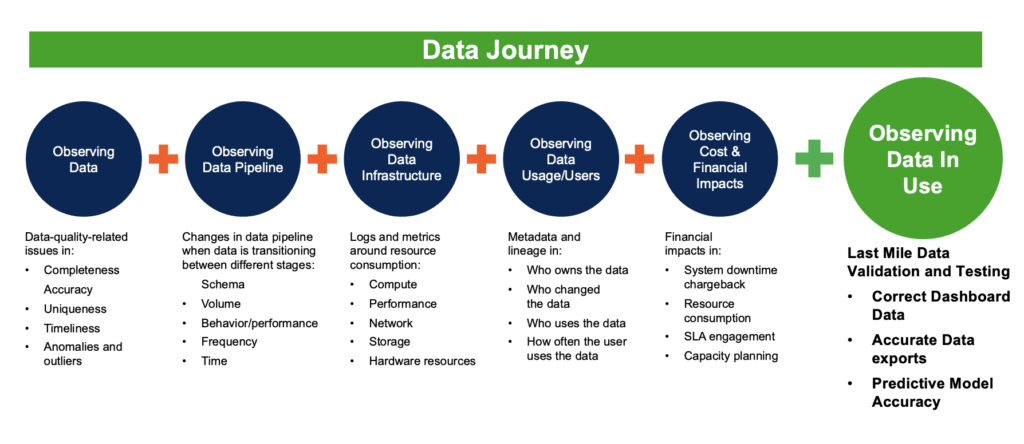
Data Observability Needs Data Journeys to Include the Last Mile of “Data in Use”
The gap in Chien’s analysis of Data Observability involves naming one of the five critical technologies as data lineage vs. a data journey. While interrelated, Data Lineage and Data Journey have distinct characteristics and functionalities within data management and analytics. They provide different types of analysis – static (Data Lineage) and dynamic (Data Journey). These analyses serve different purposes and answer questions about the data’s provenance, reliability, and current state. Data Lineage, a form of static analysis, is like a snapshot or a historical record describing data assets at a specific time. It’s primarily used to understand where data came from and its transformations. With a data catalog, data lineage can provide a comprehensive understanding of specific point-in-time content data tables or files. However, it can’t confirm if today’s dashboard can be trusted or if it has been updated with the most recent files because it represents a static analysis of the data’s past journey.
Data Lineage is static analysis for data systems. As any developer knows, static analysis examines the code without executing it. And as any developer knows, you can’t ship code based on static tests. You must dynamically test the code.
In contrast, a Data Journey offers a dynamic analysis of the live execution of data analytic tools, data, and software operations. It goes beyond the historical record provided by data lineage to include real-time data analysis in motion. Data Journey captures and analyzes the operations occurring in data analytics in real time. It can answer questions about the current state of the data that data lineage can’t. For example, a Data Journey can confirm whether today’s data is trustworthy or has been updated with the most recent files. The Data Journey represents the data’s ongoing journey, including all transformations and operations it is currently undergoing.
Monitoring and testing data in use continually is crucial. This action involves testing the results of data models for accuracy and relevance, evaluating the effectiveness of data visualizations, ensuring that data delivery mechanisms are operating optimally, and checking the data utilization to ensure it meets its intended purpose. The ‘Data in Use’ part of the five pillars of Data Journeys underscores the need for robust testing and evaluation processes throughout the ‘last mile’ of the Data Journey.
Want to learn more about Data Journeys?
- Data Journey Manifesto https://datajourneymanifesto.org/
- Why the Data Journey Manifesto? https://datakitchen.io/why-the-data-journey-manifesto/
- Five Pillars of Data Journeys https://datakitchen.io/introducing-the-five-pillars-of-data-journeys/
- Data Journey First DataOps https://datakitchen.io/data-journey-first-dataops/
- The Terms and Conditions of a Data Contract are Data Tests https://datakitchen.io/the-terms-and-conditions-of-a-data-contract-are-data-tests/
- “You Complete Me,” said Data Lineage to Data Journeys. https://datakitchen.io/you-complete-me-said-data-lineage-to-dataops-observability/