DataOps has become an essential methodology in pharmaceutical enterprise data organizations, especially for commercial operations. Companies that implement it well derive significant competitive advantage from their superior ability to manage and create value from data. They will be able to produce high-quality, on-demand insight that consistently leads to successful business decisions. DataOps is fundamentally about eliminating errors, reducing cycle time, building trust and increasing agility. Companies that do these things well will win the next business cycle.
After a decade of drug development and productization, the most critical phase in the lifecycle of a new pharmaceutical product is the product launch. The first 6-12 months in the life of a drug is critical to the drug’s lifetime revenue. A solid ramp in initial interest puts a new medicine on a trajectory to meet its lifetime sales targets. Most of the revenue is generated after the first year, but the product launch lays the foundation for later growth. A weak launch diminishes revenue prospects for the entire life of the drug. Figure 1 shows how the revenue curves diverge between a weak and robust product launch.
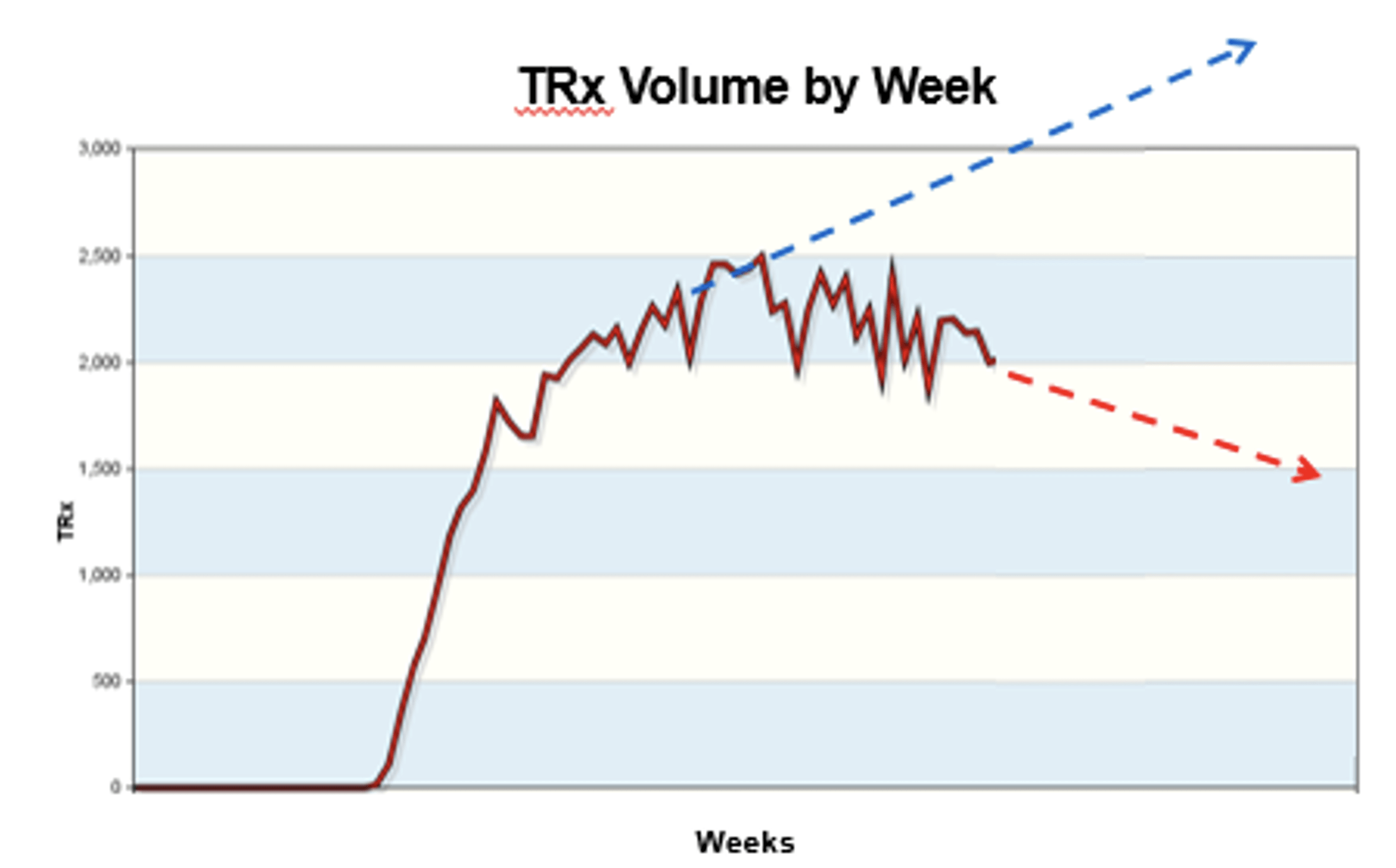
Figure 1: The first months in the product lifecycle determines its lifetime revenue.
During the product launch, everyone in the sales and marketing organizations is hyper-focused on business development. Marketing invests heavily in multi-level campaigns, primarily driven by data analytics. This analytics function is so crucial to product success that the data team often reports directly into sales and marketing.
During the critical product launch phase, the entire sales and marketing team demands analytic insights that track and improve a product’s growth trajectory. Imagine a data team of one or two dozen data professionals serving the analytics needs of hundreds of sales and marketing team members. Each user has a mission, conducting targeted campaigns or selling into a territory. They submit an endless list of requests for new data sets, dashboards, segmentations, cached data sets and nearly anything else they think will help them meet business goals. With the clock ticking on the launch calendar, there is never time to relax. The sales and marketing organizations bombard the data team with requests for analytic insights and demand immediate answers. The data team must be able to respond rapidly and with a high degree of quality and certainty to user requests.
As the analytics team and business users focus on the product trajectory and the brand’s growth, the insights must flow “fast and furious,” whether hourly, daily, weekly or monthly. As figure 2 summarizes, the data team ingests data from hundreds of internal and third-party sources.
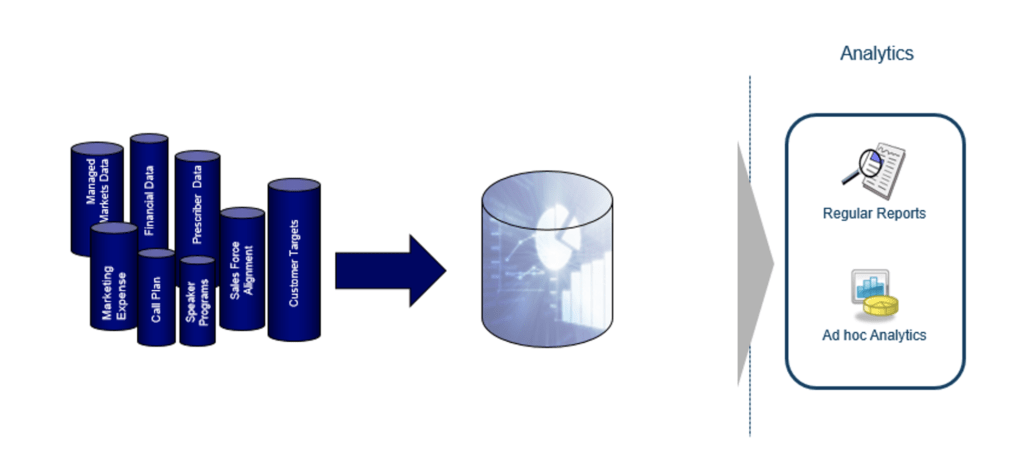
Figure 2: During the product launch, data comes from various sources and feeds into regular and ad hoc reports and analytics.
The data team must cope with the complexity of managing many data sets and the nuances associated with each data source. For example, there may be multiple lists of physicians that are not the same. There may be one million physicians in the US, but perhaps only 40,000 are marketing targets for a given drug product. Getting this standardized is vital because it affects sales compensation.
Part of the data team’s job is to make sense of data from different sources and judge whether it is fit for purpose. Figure 3 shows various data sources and stakeholders for analytics, including forecasts, stocking, sales, physician, claims, payer promotion, finance and other reports.
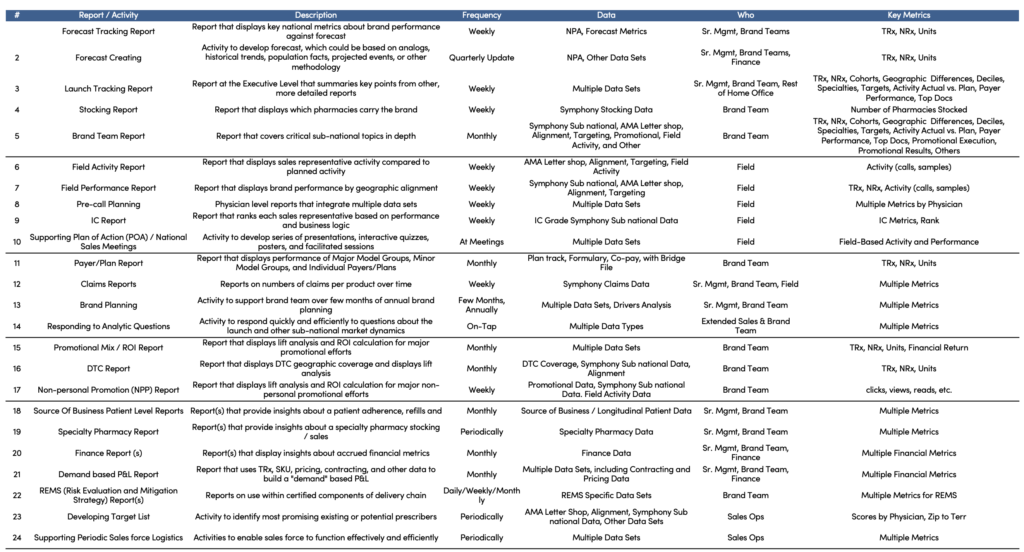
Figure 3: The vast and varied types of analytics required during the launch phase.
DataOps Success Story
The success of the billion-dollar Otezla brand illustrates how to use DataOps to effectively cope with dataset and analytics complexity. Using the DataKitchen DataOps Platform, the Otezla data analytics team designed an extraordinarily agile analytics function with a relatively small team. They mastered hundreds of data sets, serving thousands of people, with very few errors or missed SLAs (service level agreements). The Otezla team built a system with tens of thousands of automated tests checking data and analytics quality. It implemented hundreds of schema and data set changes per week without introducing errors. Arguably the most agile and effective data analytics capability in the pharmaceutical industry was accomplished cost-effectively, with a data engineering team of seven and another 10-12 data analysts. The Otezla data team designed a very efficient, rapid-response, high-quality method of working that gave great insight and made a significant material impact on the Otezla brand. Without DataOps, companies can employ hundreds of data professionals and still struggle. That’s the power of DataOps automation.
Let’s take a look at an example DataOps implementation using DataKitchen for an actual biologic launch – Figure 4. The data pipelines must contend with a high level of complexity – over seventy data sources and a variety of cadences, including daily/weekly updates and builds. Biologic data is complicated and not clean, so the DataOps Platform first profiles the data and imposes automated, rule-based quality checks. Has the data arrived on time? Is the quantity of data correct? When the tests pass, the orchestration admits the data to a data catalog.
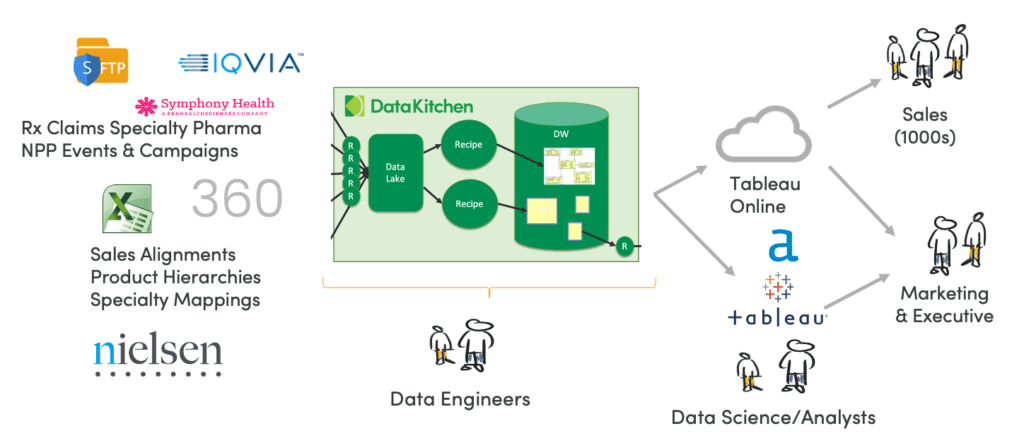
Figure 4: DataOps architecture based on the DataKitchen Platform
New data is shared with users by updating reporting schema several times a day. The architecture takes purpose-built data warehouses/marts and other forms of aggregation and star views tailored to analyst requirements. When these builds are complete, notifications trigger refreshes of dashboards, tableau workbooks, and whatever standard reports the business unit requires.
The analytics supported by the DataOps Platform are extremely agile from two perspectives. The production analytics are updated several times a day using dozens of data sources arriving asynchronously. Perhaps more importantly, data engineers and scientists may change any part of the automated pipelines related to data at any time. In this case, data team members routinely made dozens of changes to the process weekly. Changes included new data sets, new schemas, new views – whatever business stakeholders required. The data organization accomplished all of this flexibility while maintaining negligible error rates because DataOps tests data at every stage of the process. DataOps observability catches errors, so they never reach the consumers of analytics.
The DataKitchen DataOps Platform implements automation that replaces an army of people who previously executed manual tests, checklists and procedures. The team redeploys its newly freed resources on projects that create analytics that fulfill business requirements. The DataOps Platform allows commercial pharma analytics teams to produce more insight, faster and at the speed that the customers and business demand. Some features and benefits of the DataKitchen DataOps Platform are shown in the table below.
When the processes that act upon data are designed for rapid refresh and rapid-response to analytics questions, the data team can achieve a much faster time-to-insight and lower cost of implementation for ad hoc requests. DataOps automation replaces the non-value-add work performed by the data team and the outside dollars spent on consultants with an automated framework that executes efficiently and at a high level of quality. Focusing on the processes that operate on data enables the team to automate workflows and build a factory that produces insights.
The DataOps Platform does not replace a data lake or the data hub. It is an automation layer that spans the entire existing toolchain. If you are an Informatica shop or run Snowflake or Databricks – DataKitchen works alongside data ecosystem tools and integrates them into the end-to-end data lifecycle.
The DataOps process-centric approach takes the view that the processes that act upon data are as important as the data itself. Neglecting those processes opens an organization up to delays, errors and unplanned downtime. Embracing the process-oriented approach to data analytics leads to ever faster and more flexible analytics development and operations with virtually zero downtime.
DataOps Is the “New Normal” in Pharma
Otezla employed DataOps automation with DataKitchen to enable extremely fast changes to support investigative analytics and high-quality production deliverables. They were able to build analytics consistently across dozens of product teams. The Otezla team deployed pipelines at scale across a large number of people and spanning a multitude of toolchains.
DataOps is about quality assurance, automation, reusability, and repeatability. It strives for analytics agility which leads to business agility. It employs automation to streamline resources while increasing productivity. Otezla found that when the analytics team works rapidly and accurately, they can better support decision-makers. It’s that simple.
Curious how DataOps can improve Pharma R&D operations? Visit our blog, Accelerating Drug Discovery and Development with DataOps.